Jing HE, Quancheng LIU, Zhonggang XIONG, Linyu CHEN. Determination of copper content in raw ores via laser-induced breakdown spectroscopy with generalized linear model[J]. Infrared and Laser Engineering, 2025, 54(2): 20240455

Search by keywords or author
- Infrared and Laser Engineering
- Vol. 54, Issue 2, 20240455 (2025)
Note: This section is automatically generated by AI . The website and platform operators shall not be liable for any commercial or legal consequences arising from your use of AI generated content on this website. Please be aware of this.
Abstract
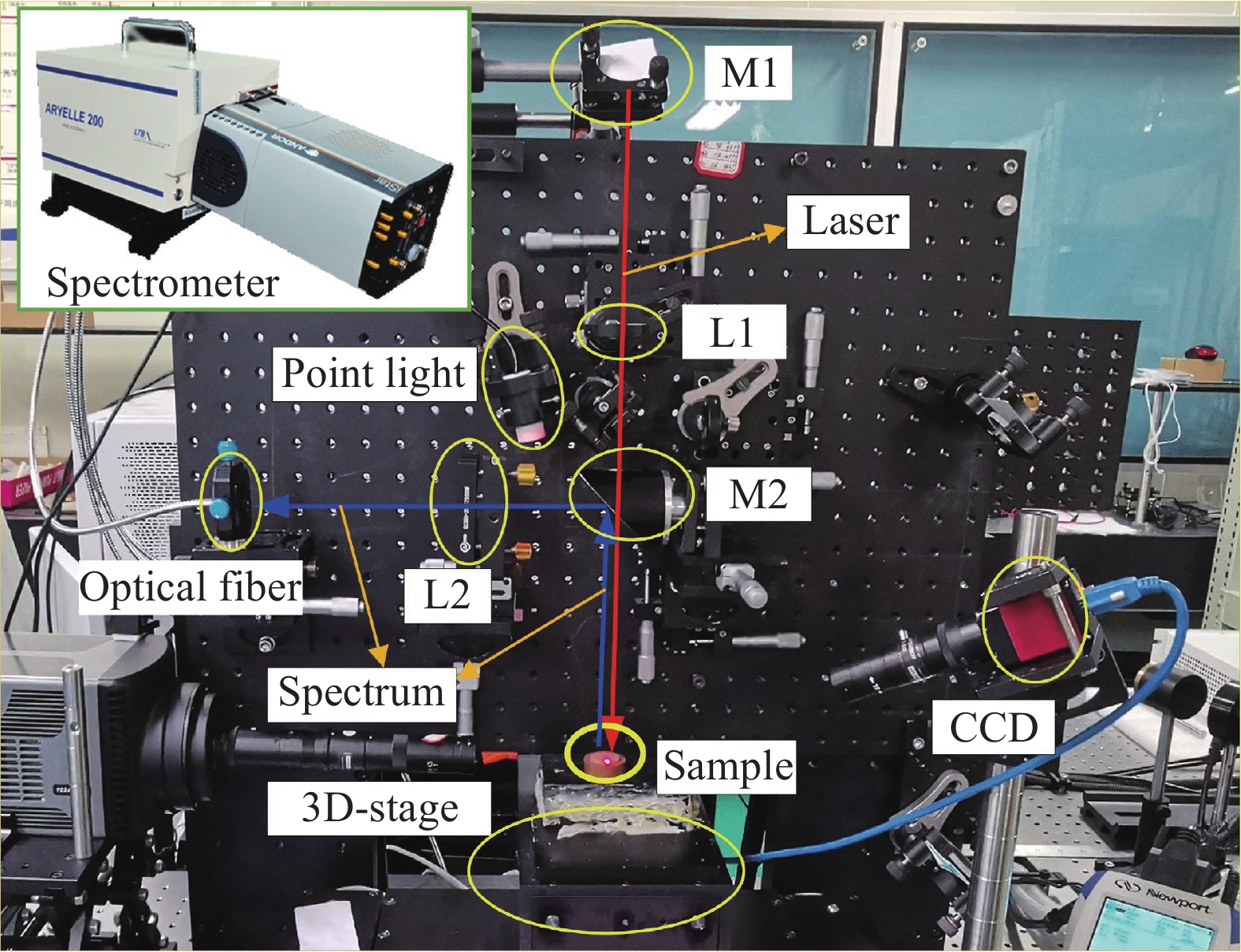
Set citation alerts for the article
Please enter your email address