Jing HE, Quancheng LIU, Zhonggang XIONG, Linyu CHEN. Determination of copper content in raw ores via laser-induced breakdown spectroscopy with generalized linear model[J]. Infrared and Laser Engineering, 2025, 54(2): 20240455

Search by keywords or author
- Infrared and Laser Engineering
- Vol. 54, Issue 2, 20240455 (2025)
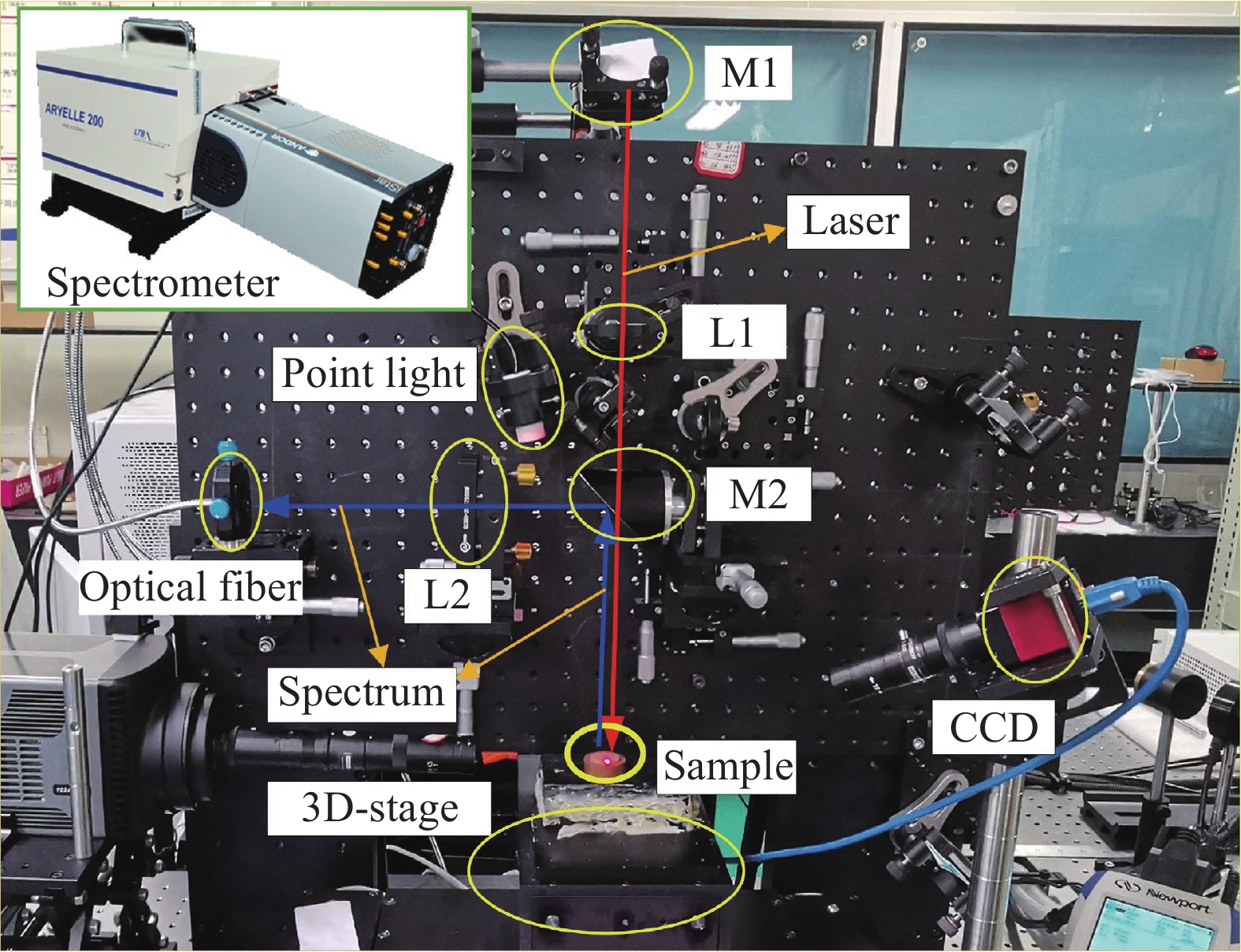
Fig. 1. Schematic of LIBS experimental setup

Fig. 2. Tablet images of nine kinds of ore/concentrate samples
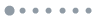
Fig. 3. Typical spectra of nine kinds of copper ore/concentrate
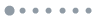
Fig. 4. Variation of R 2 in the training and test sets with α
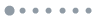
Fig. 5. The number of selected Cu lines with α
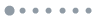
Fig. 6. Predicted and validated results of Elastic Net
|
Table 1. The spectra sets and speciality of copper ore/concentrate
|
Table 2. Performance comparison between eight models
|
Table 3. Fitting MSE of Leave-one-out method
|
Table 4. Validation MSE of Leave-one-out method
|
Table 5. Paired T-Test
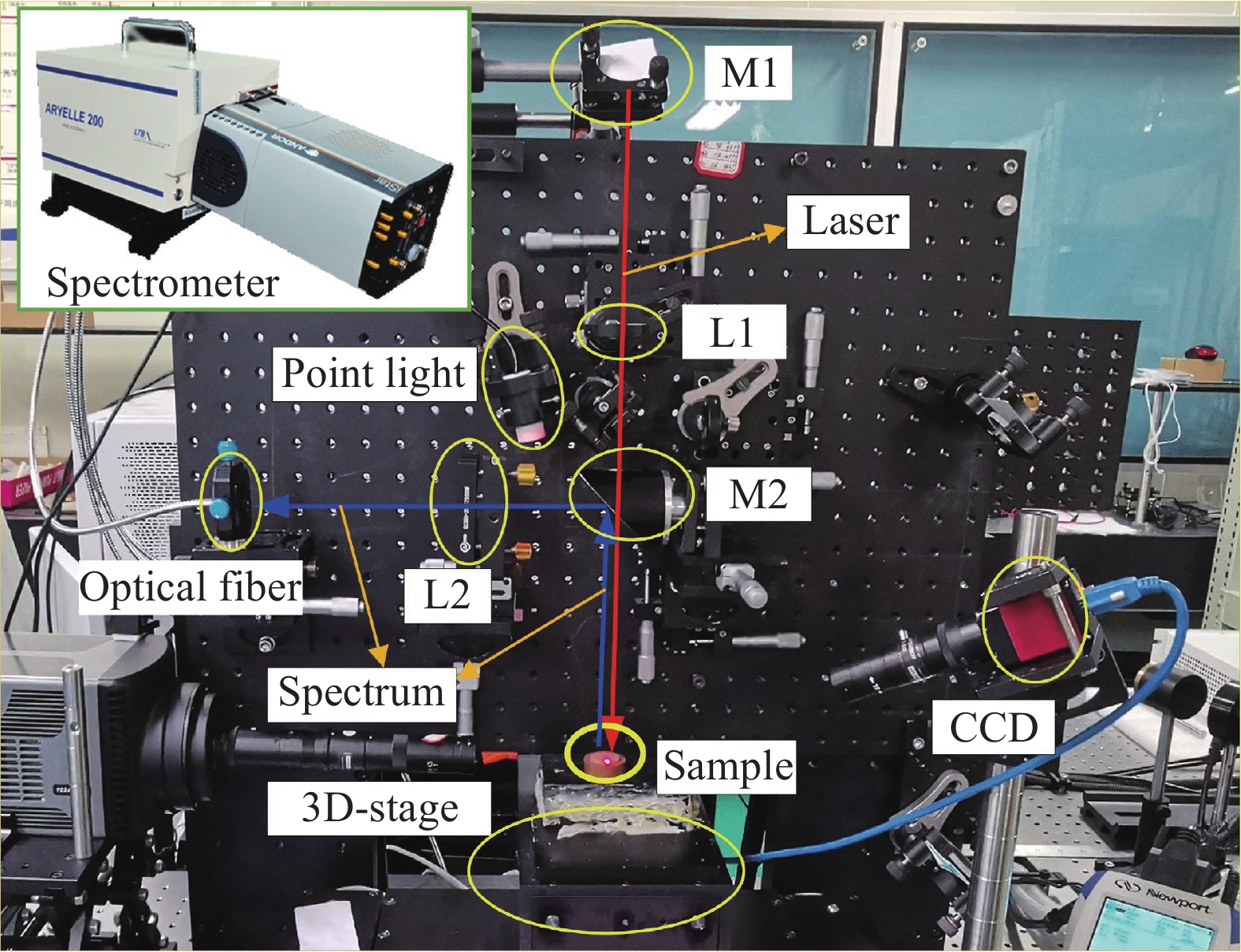
Set citation alerts for the article
Please enter your email address