Hang Su, Yanping He, Baoli Li, Haitao Luan, Min Gu, Xinyuan Fang, "Teacher-student learning of generative adversarial network-guided diffractive neural networks for visual tracking and imaging," Adv. Photon. Nexus 3, 066010 (2024)

Search by keywords or author
- Advanced Photonics Nexus
- Vol. 3, Issue 6, 066010 (2024)
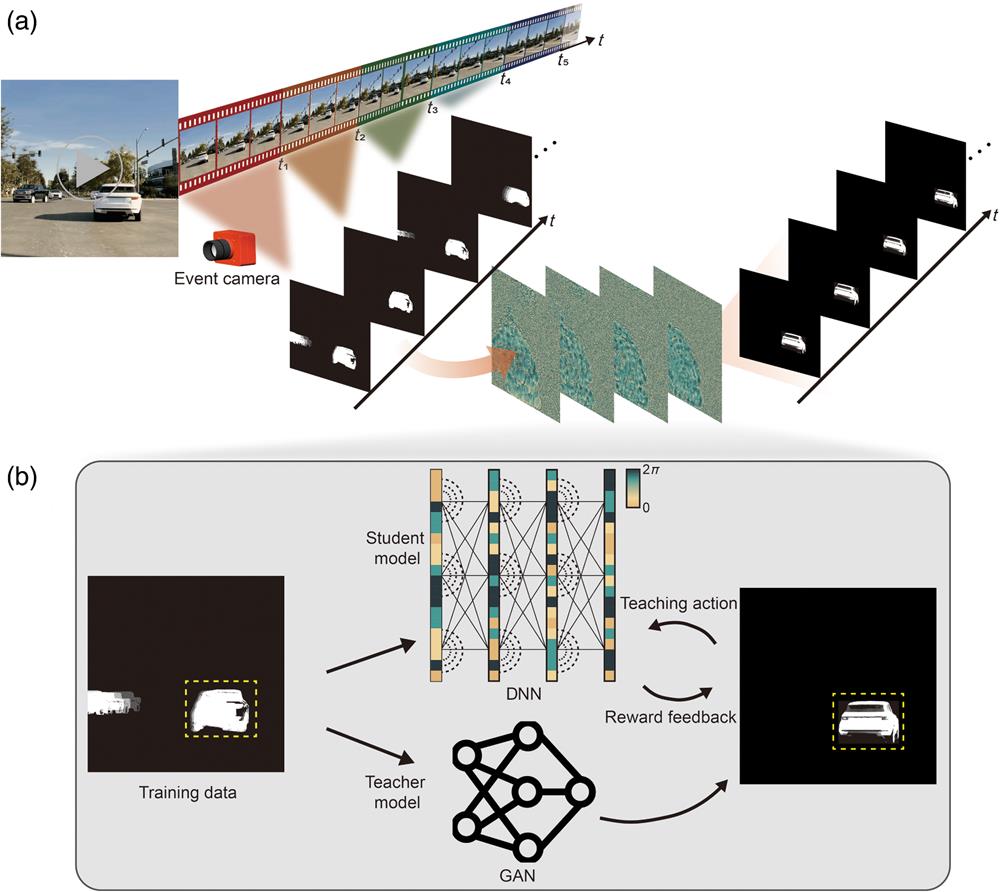
Fig. 1. The overall working principle of the GAN-guided DNN. (a) GAN-guided DNN for visual tracking and imaging of the interested moving target. (b) The training process of the GAN-guided DNN.

Fig. 2. The process of training the GAN-based teacher model, which involves acquiring datasets and optimizing models. (a) The principle of input dataset acquisition using the event-based camera. (b) The architecture of the GAN-based teacher model.
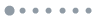
Fig. 3. Simulation results of GAN-guided DNN. (a) Examples of training results for the visual tracking and imaging of the target car. (b) The phase profiles of diffractive layers after deep learning-based optimization. (c) The PSNR and SSIM values with different input images.
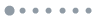
Fig. 4. The GAN-guided DNN trained and tested with different numbers of diffractive layers. (a) The performance of the GAN-guided DNN with different numbers of diffractive layers ( ) for the scenario with just a pedestrian and some static objects. (b) The SSIM and PSNR values with different input images.
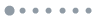
Fig. 5. Experimental demonstration of the visual tracking using a GAN-guided DNN. (a) Schematic diagram of the experimental setup and phase used in the experiment (layer 1 and layer 2 are loaded on SLM 1 and SLM 2, respectively). HWP, half-wave plate; PBS, polarization beam splitter; QWP, quarter-wave plate; BS, beam splitter; SLM, spatial light modulator. (b) Simulation and experimental results of visual tracking and imaging of the target airplane in a scenario involving airplanes and missiles. (c) The SSIM and PSNR values of the simulation and experimental results with different input images.
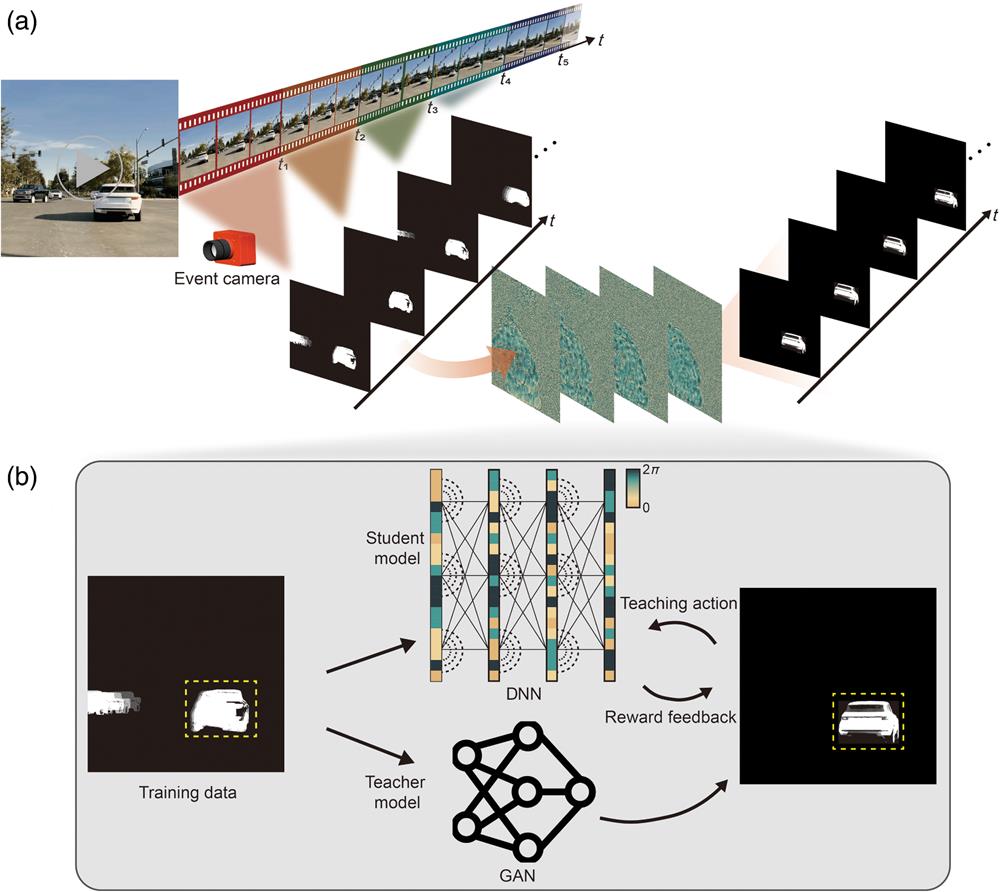
Set citation alerts for the article
Please enter your email address