
Search by keywords or author
- Advanced Photonics Nexus
- Vol. 3, Issue 6, 066010 (2024)
Abstract
Keywords
Supplementary Materials
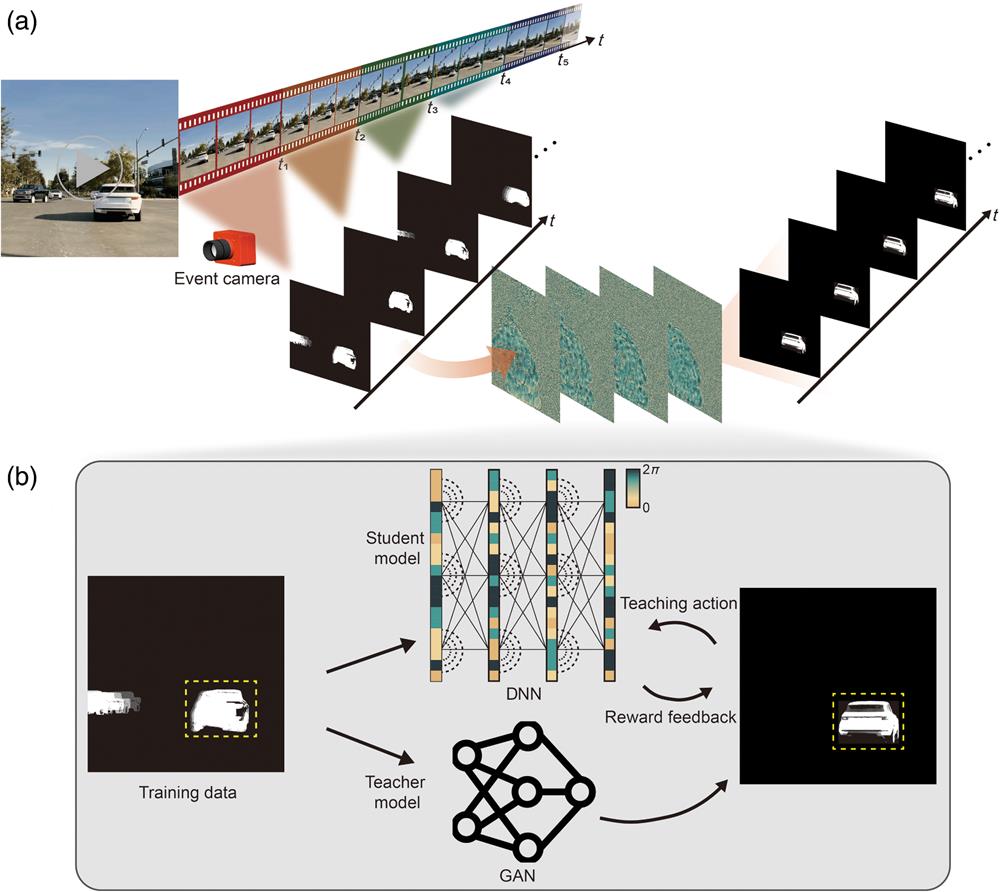
Hang Su, Yanping He, Baoli Li, Haitao Luan, Min Gu, Xinyuan Fang, "Teacher-student learning of generative adversarial network-guided diffractive neural networks for visual tracking and imaging," Adv. Photon. Nexus 3, 066010 (2024)
Download Citation
Set citation alerts for the article
Please enter your email address