Fanna Meng, ZouYongjia, Yang Cao, Jin Lü, Hongfei Yu. Stereo Matching Algorithm Based on Adaptive Spatial Convolution[J]. Laser & Optoelectronics Progress, 2025, 62(8): 0815012

Search by keywords or author
- Laser & Optoelectronics Progress
- Vol. 62, Issue 8, 0815012 (2025)
Note: This section is automatically generated by AI . The website and platform operators shall not be liable for any commercial or legal consequences arising from your use of AI generated content on this website. Please be aware of this.
Abstract
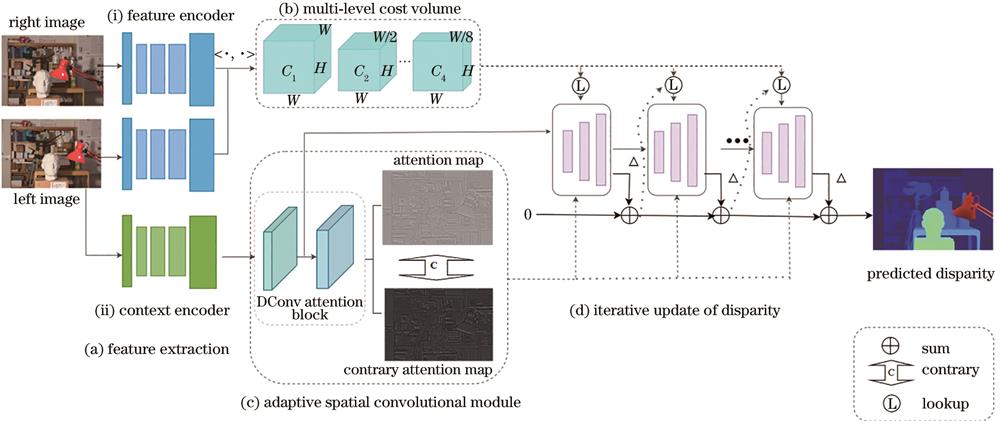
Set citation alerts for the article
Please enter your email address