Fanna Meng, ZouYongjia, Yang Cao, Jin Lü, Hongfei Yu. Stereo Matching Algorithm Based on Adaptive Spatial Convolution[J]. Laser & Optoelectronics Progress, 2025, 62(8): 0815012

Search by keywords or author
- Laser & Optoelectronics Progress
- Vol. 62, Issue 8, 0815012 (2025)
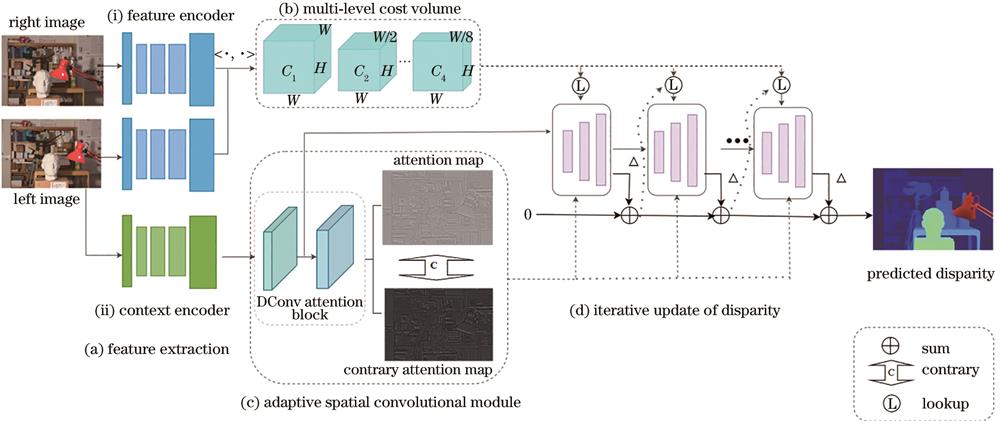
Fig. 1. Overall framework of network
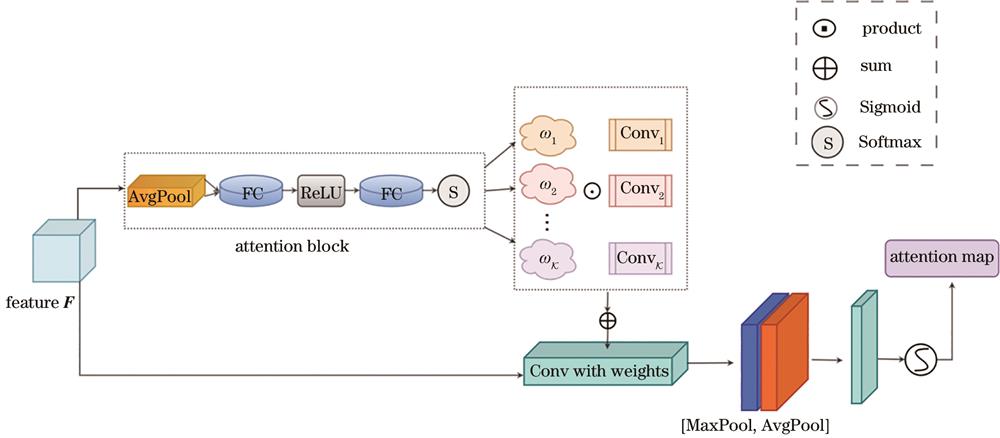
Fig. 2. Adaptive spatial convolution module
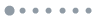
Fig. 3. Comparison of disparity maps in KITTI reflection areas. (a) Left view; (b) benchmark algorithm; (c) ours
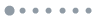
Fig. 4. Comparison of disparity maps in KITTI occlusion regions. (a) Left view; (b) benchmark algorithm; (c) ours
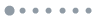
Fig. 5. Visualization results comparison on Middlebury dataset. (a) Left view; (b) benchmark algorithm; (c) ours
|
Table 1. Pre-training model parameters
|
Table 2. Ablation experiment
|
Table 3. Only ablation analysis of changing ASCT convolutional kernel number
|
Table 4. Only ablation analysis of changing GRU convolutional kernel size
|
Table 5. Quantitative results with existing mainstream stereo matching methods on KITTI 2015 test
|
Table 6. Quantitative results with existing mainstream stereo matching methods on KITTI 2012 test
|
Table 7. Quantitative results with mainstream stereo matching methods on Scene Flow, ETH3D and Middlebury
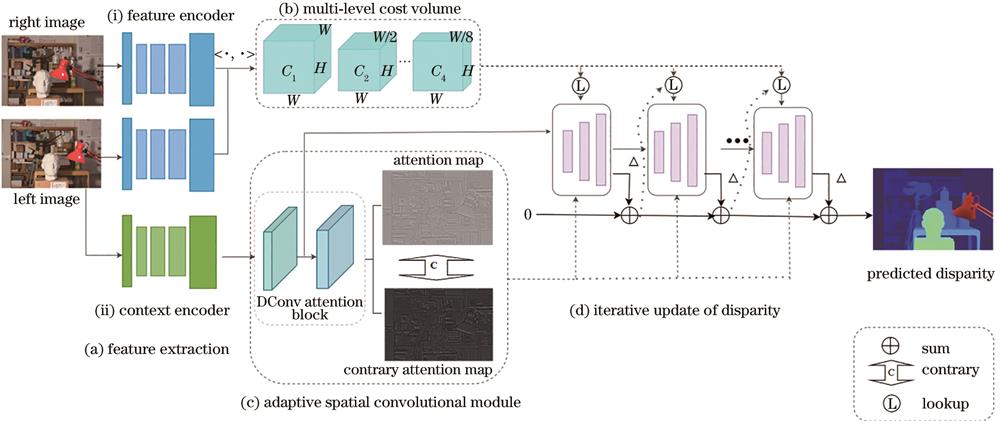
Set citation alerts for the article
Please enter your email address